Anderson A.C. Alves, PhD – Assistant Professor, Department of Animal and Dairy Science (e: alvesand@uga.edu, p: 706-542-9105; web: www.alveslab.org0
Monitoring feeding and activity behavior in dairy and beef cattle has gained significant attention due to its strong association with productivity, efficiency, and animal welfare. Continuous behavioral monitoring in lactating cows allows farmers to detect early signs of illness, make informed breeding decisions, optimize nutrition, and improve heat abatement strategies. It also helps identify stress levels and social interactions, leading to better overall herd management.
For research purposes, behavior monitoring was, not long ago, a task assigned to graduate students, much to their dismay. Hours of staring at cows and scribbling notes were as exhausting as they sound, not to mention impractical for commercial dairy farms. Fortunately, Precision Livestock Farming (PLF) technologies have emerged as reliable alternatives, offering continuous and automated monitoring solutions. Various systems are available on the market, including ear tags, collars, and ankle bracelets, which can provide accurate individual activity data. While these wearable sensors offer valuable insights, they require installation on each animal, can be costly, and may necessitate barn-specific modifications. Additionally, integrating multiple sensors from different companies is often complicated. If cows were to wear all the available technologies, they might need “extra ears and legs.”
A promising and cost-effective alternative is the use of monitoring cameras. Unlike wearable sensors, cameras are non-invasive, do not require attachment to the animals, and can simultaneously monitor multiple individuals and indicators. However, extracting meaningful insights from video footage requires advanced algorithms capable of accurately interpreting animal behaviors. This is where Computer Vision (CV) comes in, a research field that enables computers to “see” and interpret images and videos through advanced algorithms. With rapid advancements in Artificial Intelligence(AI), CV efficiency has improved dramatically in recent years.
Dr. Alves’ research group at the University of Georgia (UGA) has been actively developing user-friendly AI solutions for dairy farmers. Their goal is to provide affordable technologies that help optimize management decisions in dairy production systems. For example, the team has been working on a camera-based system to monitor drinking, feeding, standing, and resting behaviors in dairy cows. Their computer vision system (CVS) has demonstrated the ability to accurately classify behaviors at the group level. As shown in Figure 1, the system can identify cows, draw bounding boxes around them, and assign behavioral labels with high precision. This approach is particularly useful for tracking overall herd behavior, as cows tend to follow similar patterns of resting, feeding, and activity regardless of the farm.
One of the main advantages of wearable sensors is their ability to provide data at the individual level. Farmers can use this information to identify which specific cow is sick, in heat, or under stress, just to name a few examples. On the other hand, the CVS described above cannot attain the same task since it does not identify which animal is performing each behavior. Allowing CVS to recognize each animal individually is tricky but possible, with different strategies being proposed.
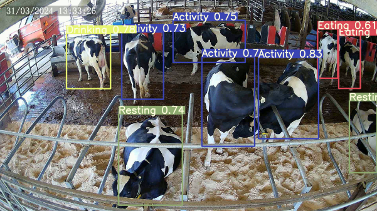
Figure 1. Predicted vs. observed total time at different behaviors according to different day periods. The predicted behavior durations were computed in minutes using a computer vision-based approach for hourly intervals: 09:00-10:00 (morning), 13:00-14:00 (afternoon), and 20:00 to 21:00 (night).
The UGA team has been developing innovative strategies to overcome this challenge. Their latest advancements allow the system to track individual cows with significantly reduced data collection and labeling efforts. Instead of requiring thousands of labeled images, their modified model needs only about 20 labeled images per animal per behavior, making the process much more efficient. Figure 2 illustrates the strong agreement between real-time behavioral observations and the system’s automated monitoring at the individual level, reinforcing its accuracy.
While this system is not perfect, cost is a critical factor to consider. Wearable sensors typically cost around $200 per cow, excluding maintenance fees. For a pen with 21 cows (as in Figure 1), the initial investment would be approximately $4,200. In contrast, a CVS setup requires only a $100 surveillance camera and a $300 small computer, plus internet access, roughly ten times less than the cost of wearable sensors.
Wearable sensors are and will remain a valuable technology for dairy farmers, but progress in computer vision presents an exciting, scalable, and cost-effective alternative. These advancements pave the way for broader adoption of non-invasive, automated monitoring systems that support precision dairy farming. As research continues, AI-powered vision systems will become increasingly viable, offering farmers powerful new tools to optimize herd management and improve animal welfare. Animal monitoring could be achieved at a fraction of the cost and without turning cows into walking tech hubs.
For more information on our team’s work, please feel free to reach out via email, phone, or on our website.